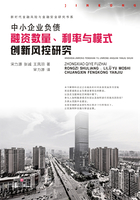
1.3 Model Design
1.3.1 Data Sources and Model Design
The data used in the research are from the monthly statistical reports of enterprise profit margin and enterprise debt asset ratio in China's large-capacity industrial enterprises(the annual main business income>=20 million yuan)during 2002 to 2016. They are all obtained from the Database of China Economic Information Network, including 179 data samples of 15 years altogether. Since they take on an irregular wavy distribution(as shown in Fig. 1-1 and Fig. 1-2), data of the nearest previous month will be assigned to the very few default values in the report.

Fig. 1-1 Linear Trend Chart of L

Fig. 1-2 Linear Trend Chart of K
According to the common sense of enterprise financial management, enterprise profit margin for the term is not only influenced by the debt ratio for the term, but also relevant to the profit margin for the previous term under the current market environment. Therefore, the model can be established as follows:

Among them, L(loan rate)stands for debt ratio, K(capital rate)for profit margin, a for the debt ratio coefficient, b for the coefficient after the first difference of the profit margin, c for the constant, and t for the time.
1.3.2 Estimation and Test of the Regression Model
From the time-series charts in Fig.1-1 and Fig.1-2 it can be seen that the variables are non-stationary and they correlate to each other to some extent, thus can be further studied. Firstly, to eliminate the heteroscedasticity, get the logarithm of the variables. Fig.1-3 andFig.1-4 are Quantitle-Quantitle charts after the logarithm processing.

Fig. 1-3 LNk and Normal Distribution

Fig. 1-4 LNk and Normal Distribution
With Eviews 6.0(statistical software for economic data), apply unit root ADF test to the non-stationary time series. Apply one of the three test types to the level:in tercept, trend and intercept or none test. Results of the three types of test all show that both LNk and LNl are non-stationary time series. E. g.:It can be seen from the ADF Test result of LNl(Table 1-1)that the value of ADF test is-0.478543, bigger than the critical value of -3.145341 at the significant level of 10%. The value P is 0.9836, much bigger than 0.05. Thus, the time series is non-stationary.
Table 1-1 ADF Test Statistic of LNl

As both LNk and LNl are non-stationary time series, it is necessary to test unit root in first difference. Choose the optimal lag difference under the standard of making the values of AIC and SC smallest, and choose among the three test types:intercept, trend and intercept or nonetest. The resuts show that LNk and LNl after first difference are both stationary time series. As shown in Table 1-2:The t-statistic value of LNl is-13.27276, much smaller than the critical value of -3.468521 at the significant level of 1%. The value P is 0. Thus, it can be sated that LNl is an integrated stationary series.
Table 1-2 ADF Test Statistic of LNl after First Difference

As can be seen from Table 1-3, the T-statistic value of LNk is -12.03050, much smaller than the critical value of -4.012296 at the significance level of 1%, and the value P is 0. The LNk can also be determined as an integrated stationary series.
Table 1-3 ADF Test Statistic of LNk after First Difference

It can seen from the ADF test statistics in the above tables that both LNl, the natural logarithm of the enterprise debt asset ratio in large-capacity Chinese industrial enterprises, and LNk, the natural logarithm of the enterprise profit margin in largecapacity Chinese industrial enterprises, are first integrated series(integrated of 1). The two are in the same order, so enabling us to further carry out the cointegration test. Press the [quick]button in EVIEWS, select the item [estimate equation], input equations either with a constant or without a constant, and compare the estimation results of the two regression models, and then we can get the long-term stationary cointegration equation of LNk and LNl.
Table 1-4 Cointegration Test Statistics of LNk and LNl


1.3.3 Estimation and Test of Residuals
To ensure the accuracy of the model, it is necessary to further test the residuals and the error correction model(ecm). Following are results of the Histogram-normality test, unit root ADF test, and Corrrogram-q-statistics of the ecm.

Fig. 1-5 Histogram-Normality Test of Residuals
From Fig. 1-5 it can be seen that the residuals take on a normal distribution, and Jarque-Bera value in its Histogram-nomality test is 5741.226, with value P of 0, which is significantly bigger than the critical value at the significant level. It means that the ecm passes the Histogram-nomality test.
Table 1-5 and table 1-6 show that the t-statistic in ADF test of the residuals is-2.243612, smaller than the critical value -1.943090 at the significant level of 5%. Thus, the time series is of integrated distribution.
Table 1-5 ADF Test Statistic of Residuals

Table 1-6 Autocorrelation and Partial Correlation Test of Residuals

At the same time, results of tenth Corrrogram-Q-statistics show that the maximum critical value of autocorrelation coefficient is -0.096, while the maximum critical value of partial correlation coefficient is -0.095. The two values are both located within the dotted line part on the two sides; the maximum value of Q is 4.1941, and the minimum value of Q is 0.2946; the minimum value of Prob is 0.587, far greater than the critical value of 0.05. Therefore, the ecm also passes the ADF test and Corrrogram-q test.
1.3.4 Interpretation of the Model
Through the estimation and test of the regression model and its residuals, it can be seen that there is a stationary cointegration relationship in the regression model in both the long run and short run.

From the above regression model we can see that the logarithm function of profit margin LNktpositively correlates to the logarithm function of the debt ratio LNlt, with a correlation coefficient of 0.087399. This indicates that from both a short-term and a long-term perspective, it is OK for industrial enterprises to moderately improve their debt asset ratios. That is to say, within the scope of controllable risks, the en terprise should adopt debt financing actively to increase the enterprise's cash flow and operation vigor, so as to improve the operating performance and its profit margin. At the same time, the correlation coefficient between LNktand LNkt-1is 0.803892. That means the enterprise profit level for the term highly correlates to its profit level for the previous term, and the operating performance for the previous term directly influence the profit level for the term.
1.3.5 Numerical Simulation Analysis
According to the regression equation of the debt ratio and profit rate of industrial enterprises, Simulink function module is designed. Then through the simulation analysis of the system, the changing relationship between the debt ratio and the profit rate can be intuitively understood.
(1)Assuming that the debt ratio of industrial enterprises is a ramp function, the slope is 0.5, the initial value of the debt ratio is 0.3 and T=10, the following simulation results can be obtained:

Fig. 1-6 Simulation System with Industrial Debt Ratio as a Ramp Function
Contrast between simulations in Fig. 1-7 and Fig. 1-8 shows that when the industrial enterprise debt ratio is a slope continuous dynamic function with its slope equaling to 0.5, the output curve of the profit rate in the industrial enterprises is similar to the curve of the slope function, namely, their trends are consistent. The marginal growth rate of the profit rate curve decreases gradually with time Tincreasing, and finally tends to L shape.

Fig. 1-7 Input:with the Debt Ratio as a Ramp Function

Fig. 1-8 Output:Simulation Results of the Profit Rate
(2)Assume that the debt ratio of industrial enterprises is a sine function, Amplitude is 1, Bias is 1, and Frequency is also 1. The minimum value of debt ratio is approaching 0, the maximum is approaching 2, time T=10, we can get the following simulation results:

Fig. 1-9 Simulation System with Industrial Debt Ratio as a Sine Function
When the industrial enterprise' debt ratio is a sine continuous dynamic function, contrast between simulations in Fig. 1-10 and Fig. 1-11 shows that the fluctuation curve of its profit rate also tends to take on a relatively consistent shape. The marginal growth rates of the two curves decrease with the heightening of them, with the only difference lying in their kurtosise and skewness.

Fig. 1-10 Input:With the Debt Ratio as a Sine Function

Fig. 1-11 Output:Simulation Results of the Profit Rate
It can be seen from the simulation results with different inputs and outputs that the fluctuation curves of the debt ratio and the profit rate tend to be similar no matter what function the input value of the debt ratio is. However, the curves show certain differences regarding to slope, kurtosis, skewness, etc. The marginal growth rates decrease with the heightening and the slope increase of the curves, finally tending to be the L or V shape.