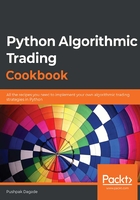
Creating a DataFrame from other formats
In this recipe, you will create DataFrame objects from other formats, such as .csv files, .json strings, and pickle files. A .csv file created using a spreadsheet application, valid JSON data received over web APIs, or valid pickle objects received over sockets can all be processed further using Python by converting them to DataFrame objects.
Loading pickled data received from untrusted sources can be unsafe. Please use read_pickle() with caution. You can find more details here: https://docs.python.org/3/library/pickle.html. If you are using this function on the pickle file created in the previous recipe, it is perfectly safe to use read_pickle().
Getting ready
Make sure you have followed the previous recipe before starting this recipe.
How to do it…
Execute the following steps for this recipe:
- Create a DataFrame object by reading a CSV file:
>>> pandas.read_csv('dataframe.csv')
We get the following output:
timestamp open high low close volume
0 2019-11-13 09:00:00 71.8075 71.8450 71.7775 71.7925 219512
1 2019-11-13 09:15:00 71.7925 71.8000 71.7800 71.7925 59252
2 2019-11-13 09:30:00 71.7925 71.8125 71.7600 71.7625 57187
3 2019-11-13 09:45:00 71.7600 71.7650 71.7350 71.7425 43048
4 2019-11-13 10:00:00 71.7425 71.7800 71.7425 71.7775 45863
5 2019-11-13 10:15:00 71.7750 71.8225 71.7700 71.8150 42460
6 2019-11-13 10:30:00 71.8150 71.8300 71.7775 71.7800 62403
7 2019-11-13 10:45:00 71.7750 71.7875 71.7475 71.7525 34090
8 2019-11-13 11:00:00 71.7525 71.7825 71.7475 71.7625 39320
9 2019-11-13 11:15:00 71.7625 71.7925 71.7600 71.7875 20190
- Create a DataFrame object by reading a JSON string:
>>> pandas.read_json("""{
"timestamp": {
"0":"13-11-2019 09:00:00", "1":"13-11-2019 09:15:00",
"2":"13-11-2019 09:30:00","3":"13-11-2019 09:45:00",
"4":"13-11-2019 10:00:00","5":"13-11-2019 10:15:00",
"6":"13-11-2019 10:30:00","7":"13-11-2019 10:45:00",
"8":"13-11-2019 11:00:00","9":"13-11-2019 11:15:00"},
"open":{
"0":71.8075,"1":71.7925,"2":71.7925,"3":71.76,
"4":71.7425,"5":71.775,"6":71.815,"7":71.775,
"8":71.7525,"9":71.7625},
"high":{
"0":71.845,"1":71.8,"2":71.8125,"3":71.765,"4":71.78,
"5":71.8225,"6":71.83,"7":71.7875,"8":71.7825,
"9":71.7925},
"low":{
"0":71.7775,"1":71.78,"2":71.76,"3":71.735,"4":71.7425,
"5":71.77,"6":71.7775,"7":71.7475,"8":71.7475,
"9":71.76},
"close":{
"0":71.7925,"1":71.7925,"2":71.7625,"3":71.7425,
"4":71.7775,"5":71.815,"6":71.78,"7":71.7525,
"8":71.7625,"9":71.7875},
"volume":{
"0":219512,"1":59252,"2":57187,"3":43048,"4":45863,
"5":42460,"6":62403,"7":34090,"8":39320,"9":20190}}
""")
We get the following output:
timestamp open high low close volume
0 2019-11-13 09:00:00 71.8075 71.8450 71.7775 71.7925 219512
1 2019-11-13 09:15:00 71.7925 71.8000 71.7800 71.7925 59252
2 2019-11-13 09:30:00 71.7925 71.8125 71.7600 71.7625 57187
3 2019-11-13 09:45:00 71.7600 71.7650 71.7350 71.7425 43048
4 2019-11-13 10:00:00 71.7425 71.7800 71.7425 71.7775 45863
5 2019-11-13 10:15:00 71.7750 71.8225 71.7700 71.8150 42460
6 2019-11-13 10:30:00 71.8150 71.8300 71.7775 71.7800 62403
7 2019-11-13 10:45:00 71.7750 71.7875 71.7475 71.7525 34090
8 2019-11-13 11:00:00 71.7525 71.7825 71.7475 71.7625 39320
9 2019-11-13 11:15:00 71.7625 71.7925 71.7600 71.7875 20190
- Create a DataFrame object by unpickling the df.pickle file:
>>> pandas.read_pickle('df.pickle')
We get the following output:
timestamp open high low close volume
0 2019-11-13 09:00:00 71.8075 71.8450 71.7775 71.7925 219512
1 2019-11-13 09:15:00 71.7925 71.8000 71.7800 71.7925 59252
2 2019-11-13 09:30:00 71.7925 71.8125 71.7600 71.7625 57187
3 2019-11-13 09:45:00 71.7600 71.7650 71.7350 71.7425 43048
4 2019-11-13 10:00:00 71.7425 71.7800 71.7425 71.7775 45863
5 2019-11-13 10:15:00 71.7750 71.8225 71.7700 71.8150 42460
6 2019-11-13 10:30:00 71.8150 71.8300 71.7775 71.7800 62403
7 2019-11-13 10:45:00 71.7750 71.7875 71.7475 71.7525 34090
8 2019-11-13 11:00:00 71.7525 71.7825 71.7475 71.7625 39320
9 2019-11-13 11:15:00 71.7625 71.7925 71.7600 71.7875 20190
How it works...
In step 1, you use the pandas.read_csv() function to create a DataFrame object from a .csv file. You pass dataframe.csv, the file path from where the .csv file should be read, as an argument. Recall, you have created dataframe.csv in step 1 of the previous recipe.
In step 2, you use the pandas.read_json() function to create a DataFrame object from a valid JSON string. You pass the JSON string from the output of step 2 in the previous recipe as an argument to this function.
In step 3, you use the pandas.read_pickle() method to create a DataFrame object from a pickle file. You pass df.pickle, the file path from where the pickle file should be read, as an argument to this function. Recall, what you created df.pickle in step 3 of the previous recipe.
If you have followed the previous recipe, the outputs for all the three steps would all be the same DataFrame object. And this would be identical to df from the previous recipe.
The methods read_csv(), read_json(), and read_pickle() can take more optional arguments than the ones shown in this recipe. Refer to the official docs for complete information on these methods.
- read_csv(): https://pandas.pydata.org/pandas-docs/stable/reference/api/pandas.read_csv.html#pandas.read_csv
- read_json(): https://pandas.pydata.org/pandas-docs/stable/reference/api/pandas.read_json.html#pandas.read_json
- read_pickle(): https://pandas.pydata.org/pandas-docs/stable/reference/api/pandas.read_pickle.html#pandas.read_pickle