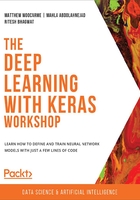
Linear Transformations
In this section, we will introduce linear transformations. Linear transformations are the backbone of modeling with ANNs. In fact, all the processes of ANN modeling can be thought of as a series of linear transformations. The working components of linear transformations are scalars, vectors, matrices, and tensors. Operations such as addition, transposition, and multiplication are performed on these components.
Scalars, Vectors, Matrices, and Tensors
Scalars, vectors, matrices, and tensors are the actual components of any deep learning model. Having a fundamental understanding of how to utilize these components, as well as the operations that can be performed on them, is key to understanding how ANNs operate. Scalars, vectors, and matrices are examples of the general entity known as a tensor, so the term tensors may be used throughout this chapter but may refer to any component. Scalars, vectors, and matrices refer to tensors with a specific number of dimensions.
The rank of a tensor is an attribute that determines the number of dimensions the tensor spans. The definitions of each are listed here:
- Scalar: They are single numbers and are an example of 0-order tensors. For instance, the temperature at any given point is a scalar field.
- Vector: Vectors are one-dimensional arrays of single numbers and are an example of first-order tensors. The velocity of a given object is an example of a vector field since it will have a speed in the two (x,y) or three (x,y,z) dimensions.
- Matrix: Matrices are rectangular arrays that span over two dimensions that consist of single numbers. They are an example of second-order tensors. An example of where matrices might be used is to store the velocity of a given object over time. One dimension of the matrix comprises the speed in the given directions, while the other matrix dimension is comprised of each given time point.
- Tensor: Tensors are the general entities that encapsulate scalars, vectors, and matrices. In general, the name is reserved for tensors of order 3 or more. An example of where tensors might be used is to store the velocity of many objects over time. One dimension of the matrix comprises the speed in the given directions, another matrix dimension is given for each given time point, and a third dimension describes the various objects.
The following diagram shows some examples of a scalar, a vector, a matrix, and a three-dimensional tensor:

Figure 2.4: A visual representation of scalars, vectors, matrices, and tensors
Tensor Addition
Tensors can be added together to create new tensors. We will use the example of matrices in this chapter, but this concept can be extended to tensors with any rank. Matrices may be added to scalars, vectors, and other matrices under certain conditions.
Two matrices may be added (or subtracted) together if they have the same shape. For such matrix-matrix addition, the resultant matrix is determined by the element-wise addition of the input matrices. The resultant matrix will, therefore, have the same shape as the two input matrices. We can define the matrix C = [cij] as the matrix sum C = A + B, where cij = aij + bij and each element in C is the sum of the same element in A and B. Matrix addition is commutative, which means that the order of A and B does not matter – A + B = B + A. Matrix addition is also associative, which means that the same result is achieved, even when the order of additions is different or even if the operation is applied more than once: A + (B + C) = (A + B) + C.
The same matrix addition principles apply for scalars, vectors, and tensors. An example of this is as follows:

Figure 2.5: An example of matrix-matrix addition
Scalars can also be added to matrices. Here, each element of the matrix is added to the scalar inpidually, as is shown in the below figure:

Figure 2.6: An example of matrix-scalar addition
It is possible to add vectors to matrices if the number of columns between the two matches each other. This is known as broadcasting.
Exercise 2.01: Performing Various Operations with Vectors, Matrices, and Tensors
Note
For the exercises and activities within this chapter, you will need to have Python 3.7, Jupyter, and NumPy installed on your system. All the exercises and activities will be primarily developed in Jupyter notebooks. It is recommended to keep a separate notebook for different assignments unless advised not to. Use the following link to download them from this book's GitHub repository: https://packt.live/2vpc9rO.
In this exercise, we are going to demonstrate how to create and work with vectors, matrices, and tensors within Python. We will assume that you have some familiarity with scalars. This can all be achieved with the NumPy library using the array and matrix functions. Tensors of any rank can be created with the NumPy array function.
Before you begin, you should set up the files and folders for this chapter in your working directory using a similar structure and naming convention as you did in the previous chapter. You can verify your folder structure by comparing it to the GitHub repository, linked above.
Follow these steps to perform this exercise:
- Open Jupyter Notebook to implement this exercise. Import the necessary dependency. Create a one-dimensional array, or a vector, as follows:
import numpy as np
vec1 = np.array([1, 2, 3, 4, 5, 6, 7, 8, 9, 10])
vec1
The preceding code produces the following output:
array([ 1, 2, 3, 4, 5, 6, 7, 8, 9, 10])
- Create a two-dimensional array, or matrix, with the array function:
mat1 = np.array([[1, 2, 3], [4, 5, 6], [7, 8, 9], [10, 11, 12]])
mat1
The preceding code produces the following output:
array([[ 1, 2, 3],
[ 4, 5, 6],
[ 7, 8, 9],
[10, 11, 12]])
- Use the matrix function to create matrices, which will show a similar output:
mat2 = np.matrix([[1, 2, 3], [4, 5, 6], \
[7, 8, 9], [10, 11, 12]])
- Create a three-dimensional array, or tensor, using the array function:
ten1 = np.array([[[1, 2, 3], [4, 5, 6]], \
[[7, 8, 9], [10, 11, 12]]])
ten1
The preceding code produces the following output:
array([[[ 1, 2, 3],
[ 4, 5, 6],
[[ 7, 8, 9],
[10, 11, 12]]])
- Determining the shape of a given vector, matrix, or tensor is important since certain operations, such as addition and multiplication, can only be applied to components of certain shapes. The shape of an n-dimensional array can be determined using the shape method. Write the following code to determine the shape of vec1:
vec1.shape
The preceding code produces the following output:
(10, )
- Write the following code to determine the shape of mat1:
mat1.shape
The preceding code produces the following output:
(4, 3)
- Write the following code to determine the shape of ten1:
ten1.shape
The preceding code produces the following output:
(2, 2, 3)
- Create a matrix with four rows and three columns with whichever numbers you like. Print the resulting matrix to verify its shape:
mat1 = np.matrix([[1, 2, 3], [4, 5, 6], [7, 8, 9], [10, 11, 12]])
mat1
The preceding code produces the following output:
matrix([[ 1, 2, 3],
[ 4, 5, 6],
[ 7, 8, 9],
[10, 11, 12]])
- Create another matrix with four rows and three columns with whichever numbers you like. Print the resulting matrix to verify its shape:
mat2 = np.matrix([[2, 1, 4], [4, 1, 7], [4, 2, 9], [5, 21, 1]])
mat2
The preceding code produces the following output:
matrix([[ 2, 1, 4],
[ 4, 1, 7],
[ 4, 2, 9],
[ 5, 21, 1]])
- Add matrix 1 and matrix 2:
mat3 = mat1 + mat2
mat3
The preceding code produces the following output:
matrix([[ 3, 3, 7],
[ 8, 6, 13],
[ 11, 10, 18],
[ 15, 32, 13]])
- Add scalars to the arrays with the following code:
mat1 + 4
The preceding code produces the following output:
matrix([[ 5, 6, 7],
[ 8, 9, 10],
[ 11, 12, 13],
[ 14, 15, 16]])
In this exercise, we learned how to perform various operations with vectors, matrices, and tensors. We also learned how to determine the shape of the matrix.
Note
To access the source code for this specific section, please refer to https://packt.live/2NNQ7VA.
You can also run this example online at https://packt.live/3eUDtQA.
Reshaping
A tensor of any size can be reshaped as long as the number of total elements remains the same. For example, a (4x3) matrix can be reshaped into a (6x2) matrix since they both have a total of 12 elements. The rank, or number of dimensions, can also be changed in the reshaping process. For example, a (4x3) matrix can be reshaped into a (3x2x2) tensor. Here, the rank has changed from 2 to 3. The (4x3) matrix can also be reshaped into a (12x1) vector, in which the rank has changed from 2 to 1.
The following diagram illustrates tensor reshaping—on the left is a tensor with shape (4x1x3), which can be reshaped to a tensor of shape (4x3). Here, the number of elements (12) has remained constant, though the shape and rank of the tensor have changed:

Figure 2.7: Visual representation of reshaping a (4x1x3) tensor into a (4x3) tensor
Matrix Transposition
The transpose of a matrix is an operator that flips the matrix over its diagonal. When this occurs, the rows become the columns and vice versa. The transpose operation is usually denoted as a T superscript upon the matrix. Tensors of any rank can also be transposed:

Figure 2.8: A visual representation of matrix transposition
The following figure shows the matrix transposition properties of matrices A and B:

Figure 2.9: Matrix transposition properties where A and B are matrices
A square matrix (that is, a matrix with an equivalent number of rows and columns) is said to be symmetrical if the transpose of a matrix is equivalent to the original matrix.
Exercise 2.02: Matrix Reshaping and Transposition
In this exercise, we are going to demonstrate how to reshape and transpose matrices. This will become important since some operations can only be applied to components if certain tensor dimensions match. For example, tensor multiplication can only be applied if the inner dimensions of the two tensors match. Reshaping or transposing tensors is one way to modify the dimensions of the tensor to ensure that certain operations can be applied. Follow these steps to complete this exercise:
- Open a Jupyter notebook from the start menu to implement this exercise. Create a two-dimensional array with four rows and three columns, as follows:
import numpy as np
mat1 = np.array([[1, 2, 3], [4, 5, 6], [7, 8, 9], [10, 11, 12]])
mat1
This gives the following output:
array([[ 1, 2, 3],
[ 4, 5, 6],
[ 7, 8, 9],
[10, 11, 12]])
We can confirm its shape by looking at the shape of the matrix:
mat1.shape
The output is as follows:
(4, 3)
- Reshape the array so that it has three rows and four columns instead, as follows:
mat2 = np.reshape(mat1, [3,4])
mat2
The preceding code produces the following output:
array([[ 1, 2, 3, 4],
[ 5, 6, 7, 8],
[ 9, 10, 11, 12]])
- Confirm this by printing the shape of the array:
mat2.shape
The preceding code produces the following output:
(3, 4)
- Reshape the matrix into a three-dimensional array, as follows:
mat3 = np.reshape(mat1, [3,2,2])
mat3
The preceding code produces the following output:
array([[[ 1, 2],
[ 3, 4]],
[[ 5, 6],
[ 7, 8]],
[[ 9, 10],
[ 11, 12]]])
- Print the shape of the array to confirm its dimensions:
mat3.shape
The preceding code produces the following output:
(3, 2, 2)
- Reshape the matrix into a one-dimensional array, as follows:
mat4 = np.reshape(mat1, [12])
mat4
The preceding code produces the following output:
array([ 1, 2, 3, 4, 5, 6, 7, 8, 9, 10, 11, 12])
- Confirm this by printing the shape of the array:
mat4.shape
The preceding code produces the following output:
(12, )
- Taking the transpose of an array will flip it across its diagonal. For a one-dimensional array, a row-vector will be converted into a column vector and vice versa. For a two-dimensional array or matrix, each row becomes a column and vice versa. Call the transpose of an array using the T method:
mat = np.matrix([[1, 2, 3], [4, 5, 6], [7, 8, 9], [10, 11, 12]])
mat.T
The following figure shows the output of the preceding code:
Figure 2.10: Visual demonstration of the transpose function
- Check the shape of the matrix and its transpose to verify that the dimensions have changed:
mat.shape
The preceding code produces the following output:
(4, 3)
- Check the shape of the transposed matrix:
mat.T.shape
The preceding code produces the following output:
(3, 4)
- Verify the matrix elements do not match when a matrix is reshaped, and a matrix is transposed:
np.reshape(mat1, [3,4]) == mat1.T
The preceding code produces the following output:
array([[ True, False, False, False],
[False, False, False, False],
[False, False, False, True]], dtype = bool)
Here, we can see that only the first and last elements match.
In this section, we introduced some of the basic components of linear algebra, including scalars, vectors, matrices, and tensors. We also covered some basic manipulation of linear algebra components, such as addition, transposition, and reshaping. By doing so, we learned how to put these concepts into action by using functions in the NumPy library to perform these operations.
Note
To access the source code for this specific section, please refer to https://packt.live/3gqBlR0.
You can also run this example online at https://packt.live/3eYCChD.
In the next section, we will extend our understanding of linear transformations by covering one of the most important transformations related to ANNs—matrix multiplication.
Matrix Multiplication
Matrix multiplication is fundamental to neural network operations. While the rules for addition are simple and intuitive, the rules for multiplication for matrices and tensors are more complex. Matrix multiplication involves more than simple element-wise multiplication of the elements. Instead, a more complicated procedure is implemented that involves the entire row of one matrix and an entire column of the other. In this section, we will explain how multiplication works for two-dimensional tensors or matrices; however, tensors of higher orders can also be multiplied.
Given a matrix, A = [aij]m x n, and another matrix, B = [bij]n x p , the product of the two matrices is C = AB = [Cij]m x p, and each element, cij, is defined element-wise as . Note that the shape of the resultant matrix is the same as the outer dimensions of the matrix product or the number of rows of the first matrix and the number of columns of the second matrix. For the multiplication to work, the inner dimensions of the matrix product must match, or the number of columns of the first matrix and the number of columns of the second matrix.
The concept of inner and outer dimensions of matrix multiplication can be seen in the following figure:

Figure 2.11: A visual representation of the inner and outer dimensions in matrix multiplication
Unlike matrix addition, matrix multiplication is not commutative, which means that the order of the matrices in the product matters:

Figure 2.12: Matrix multiplication is non-commutative
For example, let's say we have the following two matrices:

Figure 2.13: Two matrices, A and B
One way to construct the product is to have matrix A first, multiplied by B:

Figure 2.14: Visual representation of matrix A multiplied by B
This results in a 2x2 matrix. Another way to construct the product is to have B first, multiplied by A:

Figure 2.15: Visual representation of matrix B multiplied by A
Here, we can see that the matrix that was formed from the product BA is a 3x3 matrix and is very different from the matrix that was formed from the product AB.
Scalar-matrix multiplication is much more straightforward and is simply the product of every element in the matrix multiplied by the scalar so that λA = [λaij]m x n, where λ is a scalar and A is a matrix.
In the following exercise, we will put our understanding into practice by performing matrix multiplication in Python utilizing the NumPy library.
Exercise 2.03: Matrix Multiplication
In this exercise, we are going to demonstrate how to multiply matrices together. Follow these steps to complete this exercise:
- Open a Jupyter notebook from the start menu to implement this exercise.
To demonstrate the fundamentals of matrix multiplication, begin with two matrices of the same shape:
import numpy as np
mat1 = np.array([[1, 2, 3], [4, 5, 6], \
[7, 8, 9], [10, 11, 12]])
mat2 = np.array([[2, 1, 4], [4, 1, 7], \
[4, 2, 9], [5, 21, 1]])
- Since both matrices have the same shape and they are not square, they cannot be multiplied as is, otherwise, the inner dimensions of the product won't match. One way we could resolve this is to take the transpose of one of the matrices; then, we would be able to perform the multiplication. Take the transpose of the second matrix, which would mean that a (4x3) matrix is multiplied by a (3x4) matrix. The result would be a (4x4) matrix. Perform the multiplication using the dot method:
mat1.dot(mat2.T)
The preceding code produces the following output:
array([[ 16, 27, 35, 50],
[ 37, 63, 80, 131],
[ 58, 99, 125, 212],
[ 79, 135, 170, 293]])
- Take the transpose of the first matrix, which would mean that a (3x4) matrix is multiplied by a (4x3) matrix. The result would be a (3x3) matrix:
mat1.T.dot(mat2)
The preceding code produces the following output:
array([[ 96, 229, 105],
[ 111, 254, 126],
[ 126, 279, 147]])
- Reshape one of the arrays to make sure the inner dimension of the matrix multiplication matches. For example, we can reshape the first array to make it a (3x4) matrix instead of transposing. Note that the result is not the same as it is when transposing:
np.reshape(mat1, [3,4]).dot(mat2)
The preceding code produces the following output:
array([[ 42, 93, 49],
[ 102, 193, 133],
[ 162, 293, 217]])
In this exercise, we learned how to multiply two matrices together. The same concept can be applied to tensors of all ranks, not just second-order tensors. Tensors of different ranks can even be multiplied together if their inner dimensions match.
Note
To access the source code for this specific section, please refer to https://packt.live/38p0RD7.
You can also run this example online at https://packt.live/2VYI1xZ.
The next exercise demonstrates how to multiply three-dimensional tensors together.
Exercise 2.04: Tensor Multiplication
In this exercise, we are going to apply our knowledge of matrix multiplication to higher-order tensors. Follow these steps to complete this exercise:
- Open a Jupyter notebook from the start menu to implement this exercise. Begin by creating a three-dimensional tensor using the NumPy library and the array function. Import all the necessary dependencies:
import numpy as np
mat1 = np.array([[[1, 2, 3], [4, 5, 6]], [[1, 2, 3], [4, 5, 6]]])
mat1
The preceding code produces the following output:
array([[[ 1, 2, 3],
[ 4, 5, 6],
[[ 1, 2, 3],
[ 4, 5, 6]]])
- Confirm the shape using the shape method:
mat1.shape
This tensor has the shape (2x2x3).
- Create a new three-dimensional tensor that we will be able to multiply the tensor by. Take the transpose of the original matrix:
mat2 = mat1.T
mat2
The preceding code produces the following output:
array([[[ 1, 1],
[ 4, 4]],
[[ 2, 2],
[ 5, 5]],
[[ 3, 3],
[ 6, 6]]])
- Confirm the shape using the shape method:
mat2.shape
This tensor has the shape (3x2x2).
- Take the dot product of the two matrices, as follows:
mat3 = mat2.dot(mat1)
mat3
The preceding code produces the following output:
array([[[[ 5, 7, 9],
[ 5, 7, 9]],
[[ 20, 28, 36],
[ 20, 28, 36]]],
[[[ 10, 14, 18],
[ 10, 14, 18]],
[[ 25, 35, 45],
[ 25, 35, 45]]],
[[[ 15, 21, 27],
[ 15, 21, 27]],
[[ 30, 42, 54],
[ 30, 42, 54]]]])
- Look at the shape of this resultant tensor:
mat3.shape
The preceding code produces the following output:
(3, 2, 2, 3)
Now, we have a four-dimensional tensor.
In this exercise, we learned how to perform matrix multiplication using the NumPy library in Python. While we do not have to perform matrix multiplication directly when we create ANNs with Keras, it is still useful to understand the underlying mathematics.
Note
To access the source code for this specific section, please refer to https://packt.live/31G1rLn.
You can also run this example online at https://packt.live/2AriZjn.