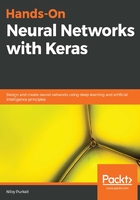
Why CNNs?
CNNs are very similar to ordinary neural networks. As we have seen in the previous chapter, neural networks are made up of neurons that have learnable weights and biases. Each neuron still computes the weighted sum of its inputs using dot products, adds a bias term, and passes it through a nonlinear equation. The network will show just one differentiable score function that will be, from raw images at one end to the class scores at other end.
And they will also have a loss function such as the softmax, or SVM on the last layer. Moreover, all the techniques that we learned ti develop neural networks will be applicable.
But then what's different with ConvNets you may ask. So the main point to note is that the ConvNet architecture explicitly assumes that the inputs that are received are all images, this assumption actually helps us to encode other properties of the architecture itself. Doing so permits the network to be more efficient from an implementation perspective, vastly reducing the number of parameters required in the network. We call a network convolutional because of the convolutional layers it has, in addition to other types of layers. Soon, we will explore how these special layers, along with a few other mathematical operations, can help computers visually comprehend the world around us.
Hence, this specific architecture of neural networks excels at a variety of visual-processing tasks, ranging from object detection, face recognition, video classification, semantic segmentation, image captioning, human pose estimation, and many, many more. These networks allow an array of computer vision tasks to be performed effectively, some critical for the advancement of our species (such as medical diagnoses), and others bordering on entertainment (superimposing a certain artistic style on a given image). Before we dive deep into its conception and contemporary implementation, it is quite useful to understand the broader scope of what we are trying to replicate, by taking a quick tour of how vision, something so complex, yet so innate to us humans, actually came about.