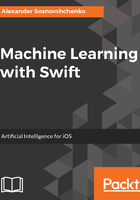
上QQ阅读APP看书,第一时间看更新
KNN pros
- It's simple to implement if you are not going for optimized versions which use advanced data structures.
- It's easy to understand and interpret. The algorithm is well studied theoretically, and much known about its mathematical properties in different settings.
- You can plug in any distance metric. This allows working with complex objects, like time series, graphs, geographical coordinates, and basically anything you can define distance metric for.
- Algorithms can be used for classification, ranking, regression (using neighbors average or weighted average), recommendations, and can even provide (a kind of) probabilistic output—what proportion of neighbors voted for this class.
- It's easy to incorporate new data in the model or remove outdated data from it. This makes KNN a good choice for online learning (see Chapter 1, Getting Started with Machine Learning) systems.