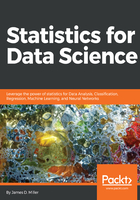
上QQ阅读APP看书,第一时间看更新
Let's move ahead
So, let's finish up this chapter with some casual (if not common sense) advice for the data developer who wants to learn statistics and transition into the world of data science.
Following are several recommendations you should consider to be resources for familiarizing yourself with the topic of statistics and data science:
- Books: Still the best way to learn! You can get very practical and detailed information (with examples) and advice from books. It's great you started with this book, but there is literally a staggering amount (and growing all the time) of written resources just waiting for you to consume.
- Google: I'm a big fan of doing internet research. You will be surprised at the quantity and quality of open source and otherwise, free software libraries, utilities, models, sample data, white papers, blogs, and so on you can find out there. A lot of it can be downloaded and used directly to educate you or even as part of an actual project or deliverable.
- LinkedIn: A very large percentage of corporate and independent recruiters use social media, and most use LinkedIn. This is an opportunity to see what types of positions are in demand and exactly what skills and experiences they require. When you see something you don't recognize, do the research to educate yourself on the topic. In addition, LinkedIn has an enormous number of groups that focus on statistics and data science. Join them all! Network with the members--even ask them direct questions. For the most part, the community is happy to help you (even if it's only to show how much they know).
- Volunteer: A great way to build skills, continue learning, and expand your statistics network is to volunteer. Check out http://www.datakind.org/get-involved. If you sign up to volunteer, they will review your skills and keep in touch with projects that are a fit for your background or you are interested in coming up.
- Internship: Experienced professionals may re-enlist as interns to test a new profession or break into a new industry (www.Wetfeet.com). Although perhaps unrealistic for anyone other than a recent college graduate, internships are available if you can afford to cut your pay (or even take no pay) for a period of time to gain some practical experience in statistics and data science. What might be more practical is interning within your own company as a data scientist apprentice role for a short period or for a particular project.
- Side projects: This is one of my favorites. Look for opportunities within your organization where statistics may be in use, and ask to sit in meetings or join calls in your own time. If that isn't possible, look for scenarios where statistics and data science might solve a problem or address an issue, and make it a pet project you work on in your spare time. These kinds of projects are low risk as there will be no deadlines, and if they don't work out at first, it's not the end of the world.
- Data: Probably one of the easiest things you can do to help your transition into statistics and data science is to get your hands on more types of data, especially unstructured data and big data. Additionally, it's always helpful to explore data from other industries or applications.
- Coursera and Kaggle: Coursera is an online website where you can take Massive Online Open Curriculum (MOOCs) courses for a fee and earn a certification, while Kaggle hosts data science contests where you can not only evaluate your abilities as you transition against other members but also get access to large, unstructured big data files that may be more like the ones you might use on an actual statistical project.
- Diversify: To add credibility to your analytic skills (since many companies are adopting numerous arrays of new tools every day) such as R, Python, SAS, Scala, (of course) SQL, and so on, you will have a significant advantage if you spend time acquiring knowledge in as many tools and technologies as you can. In addition to those mainstream data science tools, you may want to investigate some of the up-and-comers such as Paxada, MatLab, Trifacta, Google Cloud Prediction API, or Logical Glue.
- Ask a recruiter: Taking the time to develop a relationship with a recruiter early in your transformation will provide many advantages, but a trusted recruiter can pass on a list of skills that are currently in demand as well as which statistical practices are most popular. In addition, as you gain experience and confidence, a recruiter can help you focus or fine-tune your experiences towards specific opportunities that may be further out on the horizon, potentially giving you an advantage over other candidates.
- Online videos: Check out webinars and how to videos on YouTube. There are endless resources from both amateurs and professionals that you can view whenever your schedule allows.