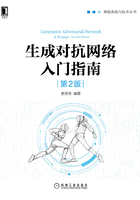
3.4 GAN的工程实践
之前几节我们了解了GAN的设计原理。但是实际是如何实现的呢?在这一节中我们会详细介绍GAN的实践方法以及代码的编写[3]。
从之前的数学推导中我们知道,我们要做的是优化下面的式子。
V = Ex~Pdata log D(x) + Ex~Pz log(1 − D(G(z))) (3-36)
计算公式中的期望值可以等价于计算真实数据分布与生成数据分布的积分,在实践中我们使用采样的方法来逼近期望值。
首先我们从前置的随机分布pg(z)中取出m个随机数{z(1), z(2),…, z(m)},其次我们再从真实数据分布pdata(x)中取出m个真实样本{x(1), x(2),…, x(m)}。我们使用平均数代替式(3-36)中的期望,公式改写如下。

在GAN的原始论文中给出了完整的伪代码(见伪代码3-2),其中θd为判别器D的参数,θg为生成器G的参数。
伪代码3-2 基础GAN的伪代码实现(其中对于判别器会迭代k次,k为超参数,大多数情况下可以使k = 1)

该伪代码每次迭代过程中的前半部分为训练判别器的过程,后半部分为训练生成器。对于判别器,我们会训练k次来更新参数θd,在论文的实验中研究者把k设为1,使得实验成本最小。生成器每次迭代中仅更新一次,如果更新多次,可能无法使得生成数据分布与真实数据分布的JS散度距离下降。
下面我们尝试使用TensorFlow来实现3.3节中GAN训练过程的可视化。
作为准备工作,我们引入对应的模块,为了支持一些旧的接口,我们在这里使用TensorFlow 2的v1兼容模块进行引入。
import numpy as np from scipy.stats import norm import tensorflow.compat.v1 as tf import matplotlib.pyplot as plt tf.disable_v2_behavior()
首先我们需要设置真实数据样本的分布,这里设置均值为3,方差为0.5的高斯分布。
class DataDistribution(object): def _init_(self): self.mu = 3 self.sigma = 0.5 def sample(self, N): samples = np.random.normal(self.mu, self.sigma, N) samples.sort() return samples
接着设定生成器的初始化分布,这里设定的是平均分布。
class GeneratorDistribution(object): def_init_(self, range): self.range = range def sample(self, N): return np.linspace(-self.range, self.range, N) + \ np.random.random(N) * 0.01
使用下面的代码设置一个最简单的线性运算函数,用于后面的生成器与判别器。
def linear(input, output_dim, scope=None, stddev=1.0): norm = tf.random_normal_initializer(stddev=stddev) const = tf.constant_initializer(0.0) with tf.variable_scope(scope or 'linear'): w = tf.get_variable('w',[input.get_shape()[1],output_dim], initializer=norm) b = tf.get_variable('b', [output_dim], initializer=const) return tf.matmul(input, w) + b
基于该线性运算函数,我们可以完成简单的生成器和判别器代码。
def generator(input, h_dim): h0 = tf.nn.softplus(linear(input, h_dim, 'g0')) h1 = linear(h0, 1, 'g1') return h1 def discriminator(input, h_dim): h0 = tf.tanh(linear(input, h_dim * 2, 'd0')) h1 = tf.tanh(linear(h0, h_dim * 2, 'd1')) h2 = tf.tanh(linear(h1, h_dim * 2, 'd2')) h3 = tf.sigmoid(linear(h2, 1, 'd3')) return h3
设置优化器,这里使用的是学习率衰减的梯度下降方法。
def optimizer(loss, var_list, initial_learning_rate): decay = 0.95 num_decay_steps = 150 batch = tf.Variable(0) learning_rate = tf.train.exponential_decay( initial_learning_rate, batch, num_decay_steps, decay, staircase=True ) optimizer = tf.train.GradientDescentOptimizer(learning_rate).minimize( loss, global_step=batch, var_list=var_list ) return optimizer
下面搭建GAN模型类的代码,除了初始化参数之外,其中核心的两个函数分别是模型的创建和模型的训练。
class GAN(object): def __init__(self, data, gen, num_steps, batch_size, log_every): self.data = data self.gen = gen self.num_steps = num_steps self.batch_size = batch_size self.log_every = log_every self.mlp_hidden_size = 4 self.learning_rate = 0.03 self._create_model() def _create_model(self): ...... def train(self): ......
创建模型。这里需要创建预训练判别器D_pre、生成器Generator和判别器Discriminator,按照之前的公式定义生成器和判别器的损失函数loss_g与loss_d以及它们的优化器opt_g与opt_d,其中D1与D2分别代表真实数据与生成数据的判别。
def _create_model(self): with tf.variable_scope('D_pre'): self.pre_input = tf.placeholder(tf.float32, shape=(self.batch_size, 1)) self.pre_labels = tf.placeholder(tf.float32, shape=(self.batch_size, 1)) D_pre = discriminator(self.pre_input, self.mlp_hidden_size) self.pre_loss = tf.reduce_mean(tf.square(D_pre - self.pre_labels)) self.pre_opt = optimizer(self.pre_loss, None, self.learning_rate) with tf.variable_scope('Generator'): self.z = tf.placeholder(tf.float32, shape=(self.batch_size, 1)) self.G = generator(self.z, self.mlp_hidden_size) with tf.variable_scope('Discriminator') as scope: self.x = tf.placeholder(tf.float32, shape=(self.batch_size, 1)) self.D1 = discriminator(self.x, self.mlp_hidden_size) scope.reuse_variables() self.D2 = discriminator(self.G, self.mlp_hidden_size) self.loss_d = tf.reduce_mean(-tf.log(self.D1) - tf.log(1 - self.D2)) self.loss_g = tf.reduce_mean(-tf.log(self.D2)) self.d_pre_params = tf.get_collection(tf.GraphKeys.TRAINABLE_VARIABLES, scope='D_pre') self.d_params = tf.get_collection(tf.GraphKeys.TRAINABLE_VARIABLES, scope='Discriminator') self.g_params = tf.get_collection(tf.GraphKeys.TRAINABLE_VARIABLES, scope='Generator') self.opt_d = optimizer(self.loss_d, self.d_params, self.learning_rate) self.opt_g = optimizer(self.loss_g, self.g_params, self.learning_rate)
训练模型的代码如下所示,首先需要预先训练判别器D_pre,然后将训练后的参数共享给判别器Discriminator。接着就可以正式训练生成器Generator与判别器Discriminator了。
def train(self): with tf.Session() as session: tf.global_variables_initializer().run() # pretraining discriminator num_pretrain_steps = 1000 for step in range(num_pretrain_steps): d = (np.random.random(self.batch_size) - 0.5) * 10.0 labels = norm.pdf(d, loc=self.data.mu, scale=self.data.sigma) pretrain_loss, _ = session.run([self.pre_loss, self.pre_opt], { self.pre_input: np.reshape(d, (self.batch_size, 1)), self.pre_labels: np.reshape(labels, (self.batch_size, 1)) }) self.weightsD = session.run(self.d_pre_params) for i, v in enumerate(self.d_params): session.run(v.assign(self.weightsD[i])) for step in range(self.num_steps): # update discriminator x = self.data.sample(self.batch_size) z = self.gen.sample(self.batch_size) loss_d, _ = session.run([self.loss_d, self.opt_d], { self.x: np.reshape(x, (self.batch_size, 1)), self.z: np.reshape(z, (self.batch_size, 1)) }) # update generator z = self.gen.sample(self.batch_size) loss_g, _ = session.run([self.loss_g, self.opt_g], { self.z: np.reshape(z, (self.batch_size, 1)) }) if step % self.log_every == 0: print('{}:{}\t{}'.format(step, loss_d, loss_g)) if step % 100 == 0 or step==0 or step == self.num_steps -1 : self._plot_distributions(session)
可视化代码如下,使用对数据进行采样的方式来展示生成数据与真实数据的分布。
def _samples(self, session, num_points=10000, num_bins=100): xs = np.linspace(-self.gen.range, self.gen.range, num_points) bins = np.linspace(-self.gen.range, self.gen.range, num_bins) # data distribution d = self.data.sample(num_points) pd, _ = np.histogram(d, bins=bins, density=True) # generated samples zs = np.linspace(-self.gen.range, self.gen.range, num_points) g = np.zeros((num_points, 1)) for i in range(num_points // self.batch_size): g[self.batch_size * i:self.batch_size * (i + 1)] = session.run(self.G, { self.z: np.reshape( zs[self.batch_size * i:self.batch_size * (i + 1)], (self.batch_size, 1) ) }) pg, _ = np.histogram(g, bins=bins, density=True) return pd, pg def _plot_distributions(self, session): pd, pg = self._samples(session) p_x = np.linspace(-self.gen.range, self.gen.range, len(pd)) f, ax = plt.subplots(1) ax.set_ylim(0, 1) plt.plot(p_x, pd, label='Real Data') plt.plot(p_x, pg, label='Generated Data') plt.title('GAN Visualization') plt.xlabel('Value') plt.ylabel('Probability Density') plt.legend() plt.show()
最后设置主函数用于运行项目,分别设置好迭代次数、批次数量以及希望展示可视化的间隔,这里的设置分别为1200、12和10。
def main(args): model = GAN( DataDistribution(), GeneratorDistribution(range=8), 1200, #num_steps 12, #batch_size 10, #log_every ) model.train()
运行过程中我们会分别看到图3-12~图3-14的几个阶段,GAN的生成器从平均分布开始会逐渐逼近最终的高斯分布,实现生成数据与真实数据分布的重合。

图3-12 GAN可视化初始阶段状态

图3-13 GAN可视化训练中状态

图3-14 GAN可视化最终状态